The Future is Now: the AI Gaming Revolution
The Cambrian Explosion in Generative AI is Only the Beginning
This is the first of a series of posts exploring the intersection of gaming and AI. This series is targeted towards founders and game developers (and the curious) to help explain the recent fuss around AI, as well as to illustrate some of its potential applications in gaming.
For more information on TIRTA Ventures, please visit our website (under construction) or reach out via LinkedIn.
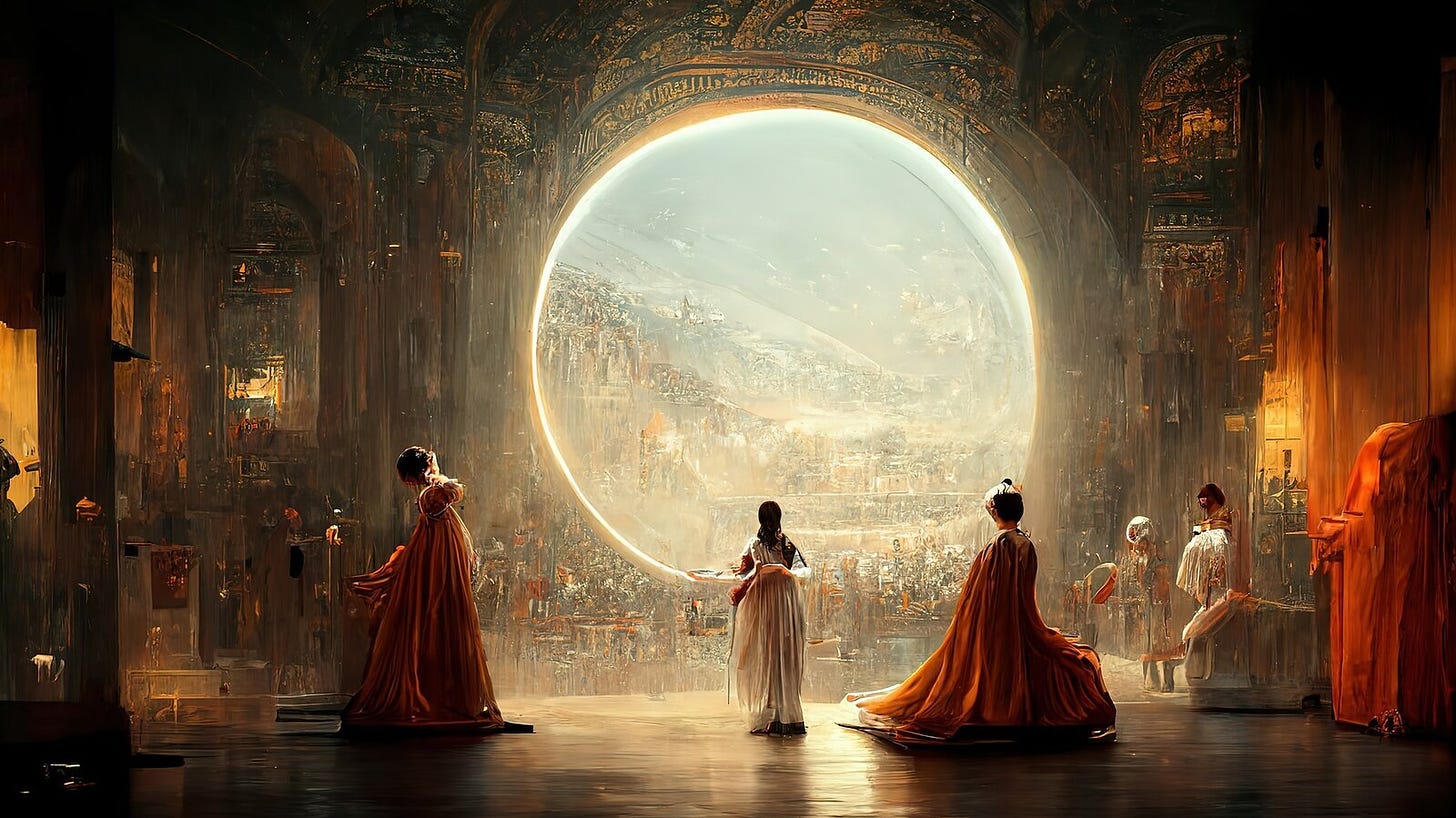
Unless you are living under a rock in some distant Tibetan monastery, you have probably been inundated over the past few months with a deluge of articles about generative AI. From AI-generated art winning competitions to AI chatbots writing college papers, it appears that 2022 is the year that AI has finally captured the popular imagination1 (not to mention the ire of the creative industry2). More recently, LENSA has overtaken social media with its fantastical avatars based on real-life people.3
A number of startups are now working on applying generative AI to more complex media formats, including audio (text-to-speech, music), 3D modeling, video, and animation.
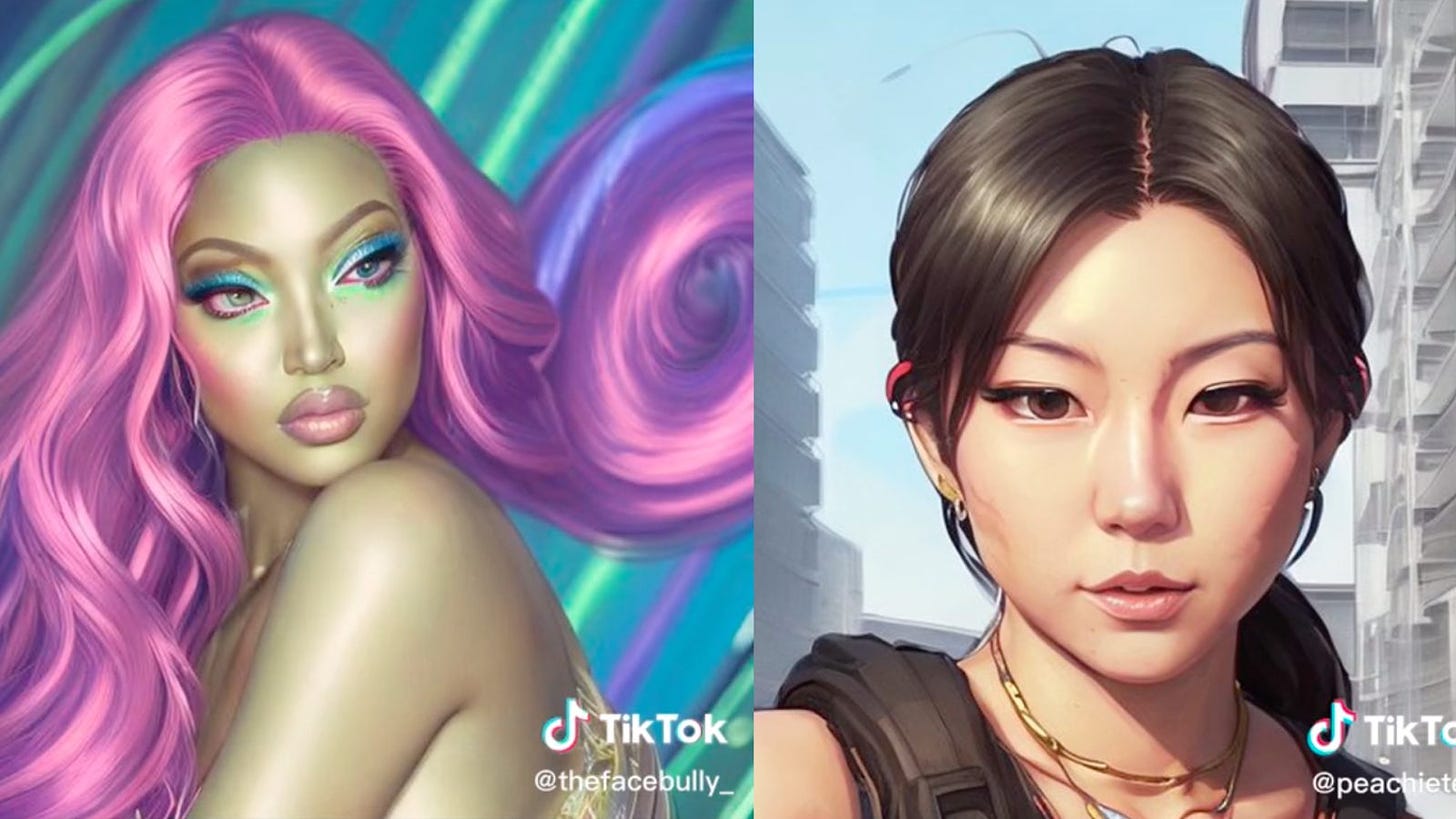
In July 2022, Midjourney launched its open beta. As an art enthusiast (with limited artistic talent), I was immediately enamored with its ability to generate high quality digital art via a simple text prompt. I felt as if I had gained a superpower4, the ability to create art that I could previously only admire from afar within the forums of DeviantArt and ArtStation. Overnight it seem, AI has bridged the gap between content creators and content consumers. As Mario Gabriele wrote eloquently in The Generalist, we are approaching a “world of infinite, customizable content”.
Defining AI
(it gets a bit technical, but bear with me)
Much of the consumer AI applications in the news recently were built using a subsect of AI technology known as deep learning (DL). DL is a sophisticated form of machine learning (ML)5 that uses artificial neural networks modeled after the human brain.
For the purpose of this post, we will be focusing on generative AI, a type of DL that underpin most of the popular tools used in AI content creation today.
Generative AI use existing content such as text, audio, and images (input) to train a neural network (or “generative model”) to create new content (output).
This training process requires very large sums of data input (GPT-3 was trained on 45 terabytes of text data6 with 175 billion parameters7), and copious amounts of computing resources (training GPT-3 required an estimated $10-20M in cloud computing costs alone). For the nerds out there, OpenAI has a good article here explaining the technology behind their generative models.
There are various techniques used to build generative models, such as generative adversarial networks, or GANs (used in the text-2-image model), transformers (GPT-3 and ChatGPT) and diffusion models (Dall-E 2, Stable Diffusion, Midjourney), a type of transformer-based generative model. I’ll leave it to the experts to provide a more detailed overview of underlying technology of generative AI.
Some of the major companies working on generative AI include:
OpenAI: SF-based company behind GPT-3, Dall-E 2, and ChatGPT (valued at >$20B as of Oct 2022 and invested by Microsoft)
Stability.AI: London-based company behind Stable Diffusion8 and Dance Diffusion (raised $101M from Coatue and Lightspeed at a $1B valuation in Oct 2022)
Google: home of the largest number of AI researchers under one roof. Developer of multiple generative models powering text-to-speech, text-to-video, and more. Google also owns Deepmind, developer of the game-playing AI AlphaGo9 and computational biology AI Alphafold
The generative AI market is projected to be worth $63B by 2028. Estimates like this should be be taken with more than a grain of salt, but it’s clear that generative AI will leave a deep mark across a number of industries, from healthcare to financial services to media. As a video game investor (and someone deeply immersed in the medium since childhood10), I am most excited about its applications in game development.
The Time is Now
Generative AI has been around for over a decade, but progress really accelerated in 2017 after researchers at Google published an influential paper describing the “transformer” architecture for neural networks, which we mentioned previously. This new technique, paired with continuous improvements in processing powers (particularly GPU performance) led to an exponential increase in model size and increases in performance11. By 2020, generative AI had begun to exceed human performance in various area, particularly in speech, language, and image recognition.
Over the past year, generative AI has seen:
Rapid improvements in underlying technology (and output quality)
In the last 12 months alone, we went from text-to-text to 2D text-to-image to text-to-video and generative music. For an an example of advances in natural-language-processing (NPL), see Google here.
Proven consumer interest
As seem in the popular adoption of generative-AI tools such as ChatGPT (crossed 1M users in under 1 week) and LENSA (grossing >$1M per day as of early Dec 202212), generative AI has tapped into an unmet consumer need - to create. Importantly, consumers are voting with their wallets.Declining costs
Currently it costs ChatGPT single-digits cents ($) per chat, over an order of magnitude more expensive than Google’s cost per search. However, costs are rapidly declining given both economies of scale as well as increased competition from open-source models.
Given the the factors above, it’s no surprise that we are now seeing a Cambrian explosion in applications built upon generative models such as GPT-3 and Stable Diffusion. Scarcely a day goes by before I see another AI media editing tool or chat app pop up in my social feed.
Why Video Games?
Among all media, videos games should reap the biggest benefit from generative AI.
The current video game industry is dominated by a handful of large developers (70% of Steam’s sales are generated by AAA/AA developers, and the console market is even more consolidated). Recent innovation in game engine and distribution (e.g. Unity, Roblox) has helped to lower the barrier to game development, but the barrier remains very high relative to other forms of media.
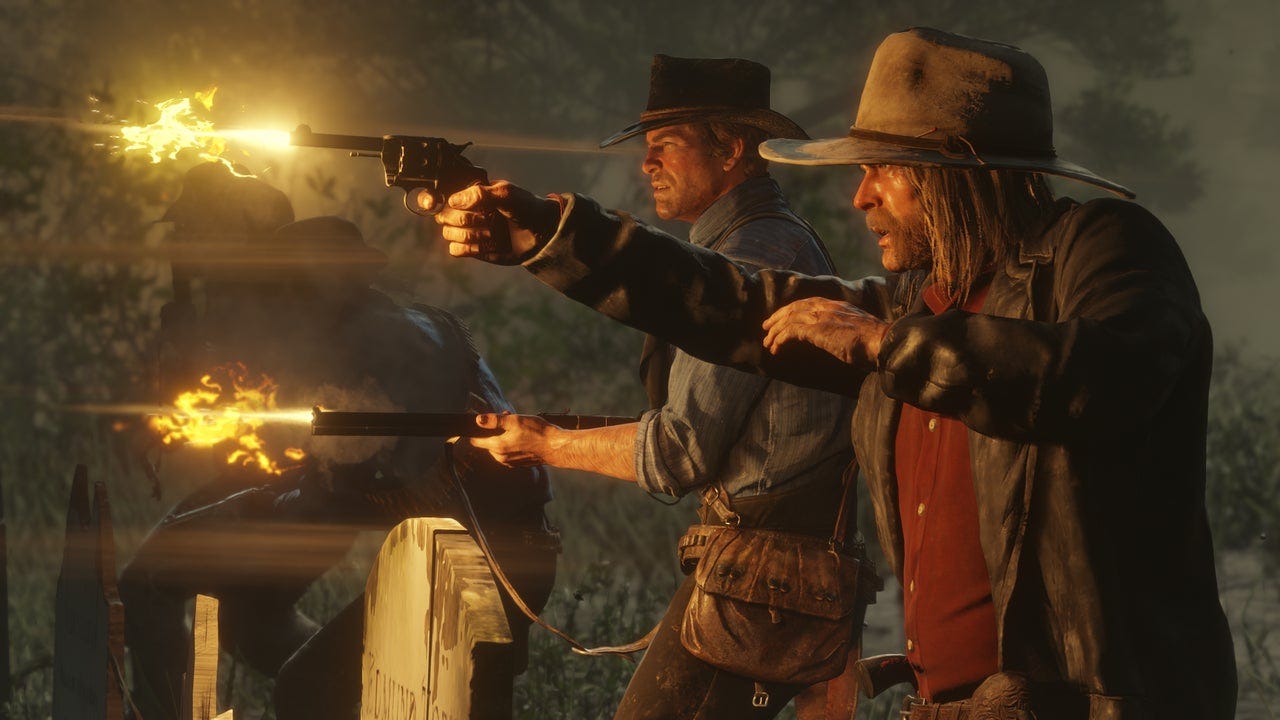
As a highly complex, digitally-native medium, it might seem self-evident that the video industry will be a major beneficiary of generative AI technology (the good folks at a16z has a great chart here), but the impact can be summarized generically as:
Cheaper: significant reduction in development cost (>50% reduction over time13)
Typical AAA games costs $150M+ to develop (and increasing) ex. marketingFaster: several orders of magnitude reduction in speed of content creation
Typical AAA/AA games has a 3+ year development cycle - developers need to predict consumer taste years ahead of product launchBetter14 (or at least indistinguishable from human-crafted)
These primary impacts create many second-order effects, including:
Smaller teams can better compete with large established developers
By lowering the cost and time required for content generation, smaller developers can use technology to narrow the gap with large AAA developers. In audio for example, there are already affordable AI tools such as murf.ai that generates high-quality dialogue in multiple languages at a fraction of the price of professional voice actors.Broader adoption of user-generated-content (“UGC”) in gaming
Despite the wide adoption of UGC across other forms of media (e.g. YouTube, TikTok, Instagram), UGC still represent a tiny fraction the gaming industry15. The Minecraft modding community and Ready Player Me's AI-powered avatar creation tool is only the tip of the spear.Enable the creation of brand new gaming experiences
Truly massive and detailed worlds will become the norm, with customized quests and dialogue for players, populated with AI NPCs, virtual companions (Replika), and personalized digital avatars. (Dare I say metaverse?)Expanding transmedia opportunities
Game developers will be able to create AI-generated videos (Synthesia) and eventually full-length movies with their in-game characters. Manga (already AI-generatable; see Google’s Giga Manga) can be turned into games and anime at a low cost.
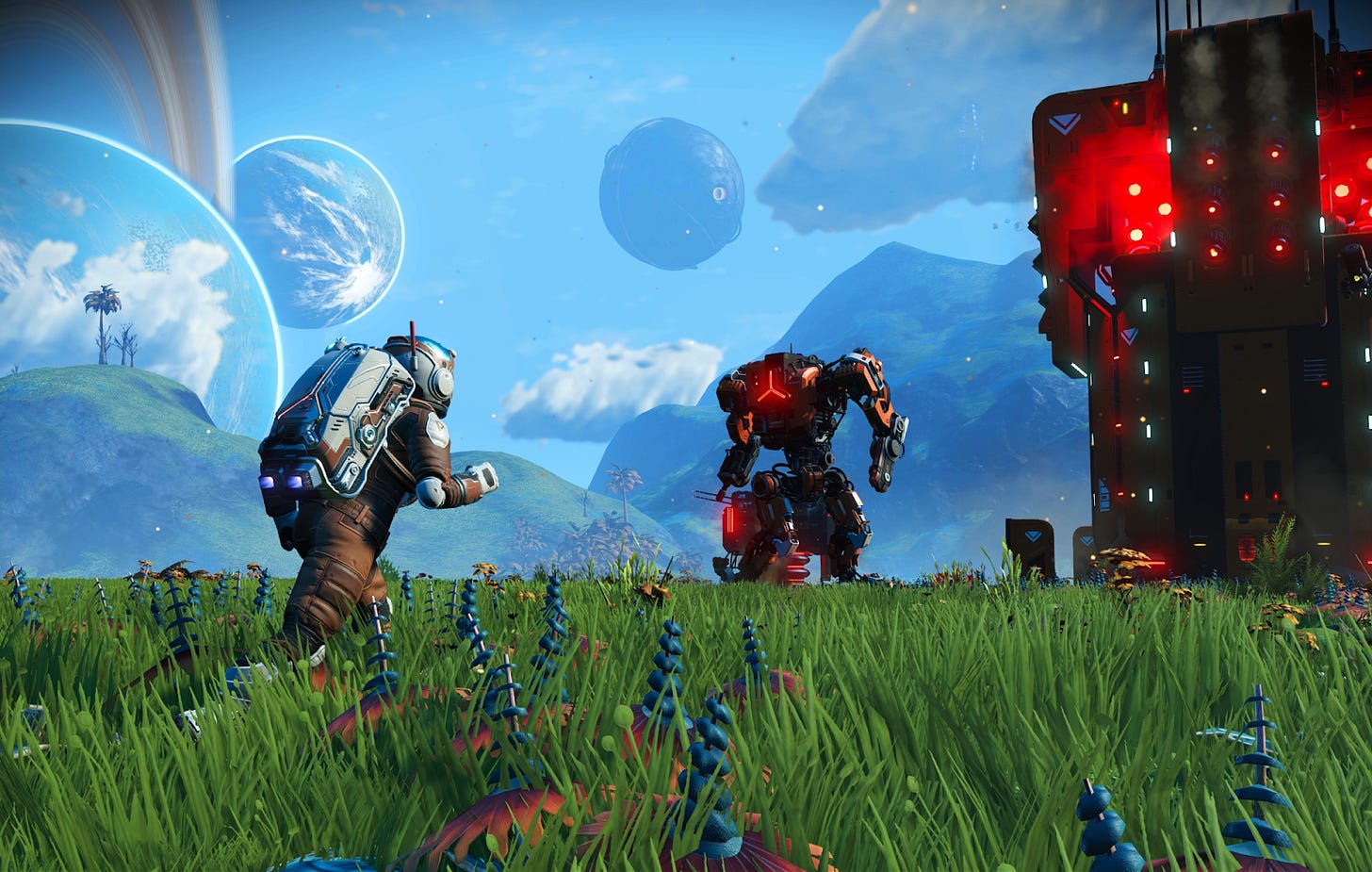
Game developers today are already using certain AI technologies, for example to remaster old games by upscaling low-res 2D textures to modern resolutions via image training (Final Fantasy VIII Remastered), but generative AI models and the applications running on them can be much more transformative.
While 2D image and text generation apps are (mostly) market ready, 3D model and video generation apps are still fairly basic (although already slick), and animating characters or building entire levels is at least several year away. It’s still early days, but the technology is progressing very rapidly.
In the meantime, developers can utilize generative AI for less ambitious tasks, such as augmenting artists and programmers with tools for prototyping, texture generation, or personalized avatar design. Games that are primarily built on text and 2D art will be the first to reap its benefits - will may soon see disruption in several genres of games, including interactive storytelling and visual novels (see Spellbrush).
Perhaps one day we will see a game that is entirely created by AI using text prompts. That day may be sooner than you think.
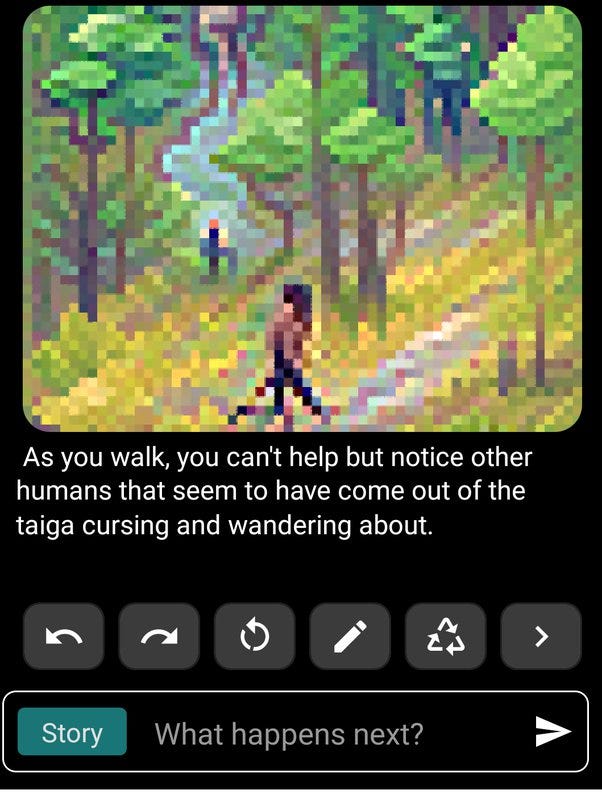
Future Posts - Stay Tuned
In no particular order:16
History of AI in Gaming
LENSA and the Future of Digital Avatars
The Gaming AI Technology Stack
Current-gen AI Applications in Gaming
Next-gen “Gaming” Applications
TAM / Analogy to the SaaS Revolution
Bottlenecks to Adoption (Enterprise & Consumer)
Who will be Disrupted?
About TIRTA Ventures
TIRTA Ventures (“TIRTA”) is newly launched VC firm based in New York City and focused on the interactive entertainment industry. TIRTA was founded by Ben Feder (former CEO of Take-Two Interactive, former President of Int’l Partnerships at Tencent Games).
TIRTA invests across content (studios), infrastructure/technology, and platforms that support the broader gaming ecosystem. We seek to bring our decades of operational experience in the gaming industry to our portfolio companies.
For more information, please visit our website (under construction).
Despite the long-held popular view that AI is within the realm of science fiction, development in the space has been ongoing since the early days of computing. From Alan Turing’s 1950 “Logic Theorists” (the first AI program), to 1986's NavLab (the first autonomous car, built at Carnegie Mellon) to 1997’s defeat of Gary Kasparov by Deep Blue in chess, innovation in AI has continued for the past 50+ years. However, despite exponential improvement in AI capability (a large part driven by Moore’s law and increases in hardware processing power), truly “human-like” AI with general intelligence and consciousness is still out of reach today.
This is a major issue for creative workers. Generative AI models are trained on vast databases of existing human-created content, much of which are used without licensing. We will attempt to address these ethical issues and potential remedies in a later post.
Despite social media companies pushing digital avatars for the past 5+ years, this might just be the “aha” moment for avatars going mainstream - more on this in a later post.
The ability to generate digital art felt to me like Neo learning kung fu via a brain-computer-interface in The Matrix.
Machine learning (ML) is about computers being able to think and act with less human intervention; deep learning (DL) is about computers learning to think using structures modeled on the human brain (artificial neural networks). While ML require less computing power, DL typically require less ongoing human intervention, and can analyze complex media and unstructured data in ways that ML cannot easily do.
GPT-3’s training data includes common crawl (webpage data), reddit, books, and Wikipedia. For reference, it is estimated that 10 terabyte can contain the entire content of the US Library of Congress, or 173M articles and books. GPT-3 was trained on 4.5x this amount!
Parameters are the values in your learning algorithm that can change independently as it learns. Parameters are affected by the choice of hyperparameters (top-level parameters dictated by humans that control the algorithm’s learning process itself). More information here.
Stable Diffusion is released by a collaboration between Stability AI, CompVis LMU (Machine Vision and Learning research group at LMU Munich), and Runway. Stable Diffusion was originally developed by CompVis LMU.
AlphaGo has since been superseded by its own progeny, AlphaZero, a generalized version of an advanced version of AlphaGo that uses reinforcement learning to train itself (without human input) to play various games (e.g. Shogi, Chess) at a highly advanced level, significantly above the level of top human players (AlphaZero has even defeated existing AIs who themselves have previously defeated top-level human players - an existential-crisis-inducing moment indeed!).
The last time I logged into the World of Warcraft in 2014, I had spent a total of 182 days inside its virtual world (>4,000 hours - that’s more than the free trial period offered in last AOL CD I received in 2005).
Generative AI models tend to scale inefficiently relative to model size (and computing cost). Model size needs to scale exponentially (an order of magnitude larger) for linear improvements in output quality (i.e. 2x).
Is it so hard to believe? The top mobile games gross $1-2B per year by selling digital goods that are largely fungible. One would think that consumers would be much more willing to pay for customized digital goods (not to mention potentially a higher paying ratio).
Based on interviews with various game developers. Content creation accounts for roughly 50% of the cost of AAA games, with the balance being programming, outsourcing, and overhead.
AI has already proven itself better than humans at in several endeavors, including playing games. Deep Blue defeated Gary Kasparov in Chess in 1997, Deepmind’s AlphaGo defeated Lee Sedol at Go in 2016, and Deepmind’s AlphaStar defeated 99.8% of players at Starcraft 2 in 2019. In healthcare, AI has proven its ability to match and even exceed humans in medical image diagnosis.
UGC has been a vibrant and influential (if niche) part of the gaming industry since the early days of gaming. Early gaming UGCs creators or “modders” were essentially white hat hackers who improved on or added to a game’s code base without the expressed permission of developers (and thus could not legally monetize). In recent years, UGC platforms such as Roblox has helped to lower the barrier to entry for game development, but the barrier remains very high for the typical player (less than 5% of Roblox’s player base are creators, and the percentage of creators who monetize is substantially lower).
And not guaranteed to publish.
Fantastic work Justin, thank you for sharing your perspectives and research!
Great article Justin... looking forward to the ability co-create in the gaming/metaverse/blockchain space!